How to get hired in data science or data analytics
A playbook
The field of data science and analytics is rich with opportunity. For seasoned professionals and newcomers alike, there are a wealth of well-paid positions that need to be filled. Nevertheless, for many the process of getting hired can be confusing and stressful, especially those who are new to the field.
So, we have teamed up with career coach and business trainer Simona Bareikė to bring you a comprehensive guide to getting hired in data science and analytics. We kick off with an overview of the current situation in terms of data science and analytics jobs, including analysis and know-how from our very own Head of Education, Giedrius Žebrauskas.
Then Simona shares her specialist insights on how to search for prospective employers and jobs. This is followed by practical guidance on reaching out to companies you like, writing applications, preparing for and performing in interviews, and passing tech challenges.
Overall, this guide is designed to be comprehensive and practical - the ultimate handbook to help you land the right data science or analytics job.
Understanding the data science employment space in 2023

Demand and supply in data science and data analytics
Demand is high and growing
Currently, there is massive demand for Data Scientists and Data Analysts. Yet, incredibly, the big data market is predicted to grow even more, up to $103 billion globally by 2027 according to Statista. This is double the size that was predicted in 2018.
In other words, the need for skilled Data Scientists and Analysts is outpacing even the most optimist forecasts. Demand is high, and is going to get even higher.
The US Bureau of Labor Statistics estimated the employment rate for Data Scientists will grow by a whopping 36% from 2021 to 2031. For context, the average growth rate for jobs overall hovers at around 5%. Demand for data professionals is also high in Europe. Harvard Business Review found that European countries make up over 80% of the cutting-edge category across business, technology and data science.
Meeting this demand
Naturally, filling so many data science and analytics positions is challenging. Governments and businesses have taken proactive steps to promote STEM jobs, especially among women, which means supply should gradually decrease.
But this is not something that will happen quickly. Anaconda’s 2022 State of Data Science report, based on 3,493 “commercial respondents” from 133 countries and regions, found that 62% are moderately, very, or extremely concerned about the potential impact of a talent shortage.
All of this is to say that, if you have been mulling over a career pivot towards data science or analytics – now is a great time to do it. There are a large number of open positions. And, despite a concerted effort to meet demand, this number is likely to remain the same or even grow.
Understanding roles in data science
Before moving on to the specifics of getting hired in data science and analytics, it is important to bust a few myths commonly held by people unfamiliar with the industry.
Afterwards, we will provide a basic breakdown of what being a Data Scientist or a Data Analyst actually entails in terms of day-to-day tasks and responsibilities.
Data Scientists are maths prodigies
OK, to be clear, data professionals do need maths, they just don't need to be maths prodigies. Working as a data professional, you will be using plenty of maths. If you are a Data Scientist working on modelling or creating algorithms, maths is one of the core skills you will be depending on day-in, day-out. "Data Science jobs often require a strong understanding of maths," explains Giedrius Žebrauskas, Head of Education at Turing College. "Skills in calculus, probabilities, statistics, and linear algebra are particularly valuable." For Data Analysts, there is a bit more room for manoeuvre in terms of your maths level. You will still be handling and manipulating large volumes of data. However, the knowledge of mathematics required will be within reach for most people who are genuinely motivated to learn.
Data professionals are, basically, wizards
To the uninitiated, data science and analytics often looks like magic. This makes Data Scientists and Analysts, surrounded by their monitors displaying arcane hieroglyphics, appear like modern day wizards. The reality, however, is quite different. Data professionals use coding, data visualisation and statistics to find patterns and trends. In the case of Data Scientists, they also use modelling and machine learning to build complex models that extract more sophisticated and subtle findings from a data set, building more intricate structures on top of simpler ones.
Data Scientists and Analysts only care about hard skills
This one is wrong for the simple reason that Data Scientists and Analysts need to not only understand data and models, but also explain them to non-specialists. They often interact with product managers, co-workers, and other stakeholders, which requires empathy and other soft skills like communication. At the end of the day, all that data – and the clever solutions based on it – has to translate into actionable insights that people can understand and use when making decisions.
A day in the life of a Data Scientist
The specific tasks a Data Scientist handles will vary widely from job to job. There is also some crossover with tasks performed by Data Analysts. The key difference is that Data Scientists will go deeper into the numbers, might look into the future as well as analysing what has already happened, and will build models and tools to facilitate this. But to give you an idea of a typical day as a Data Scientist in terms of tasks, here is a breakdown according to Anaconda's findings:

A day in the life of a Data Analyst
As a Data Analyst, you will probably be working as part of the Business Intelligence team in your company. Your role will be to act as an interpreter, taking questions or issues that are brought to you from various departments and going to the data for answers. Unlike a Data Scientist, you probably won't be creating algorithms, working with machine learning or doing modelling. Instead, you are more likely to be analysing what has already happened, and helping decision makers understand how the business is operating.
A typical day for a Data Analyst will probably include most of the following:
Receiving and understanding questions from different departments. You will need to clearly understand the business problem that is presented before you start investigating.
Gathering and cleaning data. While collecting data will usually be straightforward (though this also depends on which industry you are working in), you will also need to clean the data. This is important as it ensures no incorrect data will remain that could distort your results.
Processing the data. Here is where your skills in statistics will come into play. You will need to use tools like Excel, along with SQL, to effectively analyse the data and come up with answers to the questions you were asked. Some Data Analysts also do some programming at this stage with languages like Python, and R.
Producing reports. Using business intelligence tools like Power BI, Tableau and Looker (which are core tools for Data Analysts), you will present your findings in a manner that is accessible and clear to non-experts.
Spotting patterns. You may do some more in-depth work looking into patterns in the data that you have been working with.
Meeting your fellow analysts, and other stakeholders. You will likely need to attend meetings with your colleagues working in business intelligence and other departments, although the volume of meetings will vary from role to role.
What skills are employers looking for?
There are a wide range of skills and competences that could fall under the bracket of data science. What is more, these skills will be applied within many different industries.
According to a study conducted by 365 DataScience that looked at 1,000 LinkedIn job postings for the US, data science jobs span 50 different industries. However, the spread is uneven: 49% are in IT and tech, 14% in financial services, 11% in staffing and recruiting, 4% in industry, 3% in healthcare, and 2% in defence. It is also important to note that only about 33% of job ads specifically require a Data Science degree, and 55% of the jobs posted are entry-level.
All of which means it is hard to pin down a precise set of skills that are in demand for Data Scientists or Data Analysts.
Unsatisfactory media coverage and online resources makes this problem worse. Articles on getting a job in data science sometimes list skills in outdated technologies. Others may be promoting skills that a particular outlet is selling courses in. You will also find articles that waste readers’ time by discussing skills applicable to virtually any job. In the best case scenario, you may find an article that provides a fairly credible representation of market trends and requirements… but then gives no evidence to back up the claims being made.
The difficulty of figuring out what skills to acquire for work in data science is a problem in its own right. Not knowing what experience is actually required was singled out as one of the biggest entry barriers by as many as 20% of students considering the data science profession in Anaconda's report.
The data behind data science skills
Faced with a question like this, Luke Barousse (aka DataNerd.tech) did what any data professional would do and dived into the figures.
In fact, he painstakingly analysed almost 1 million Data Scientist, Data Analyst and Data Engineer job postings to find answers to the questions on every applicant's lips:
How many positions are there available?
What qualifications do you need?
What are the salary ranges?
And, most importantly, what skills are employers looking for?
The story of how he built an app to gather this data is fascinating in its own right. But for now, let's focus on the insights he uncovered, because they are super valuable if you want to get hired in data science or data analytics.
datanerd.tech aggregates job listings and allows users to filter them for different criteria. It is based on over 1 million job postings from across the world (not just the US where Luke is based). While there is an understandable slant towards positions in the US (it analyses over 428,000 posts from the USA), other regions are covered very well. In terms of Europe, the platform analyses over 43,000 jobs in France, 33,000 in the UK, 29,000 in Germany, 6,000 in Czechia and 2,000 in Lithuania 2,097. In terms of other regions, there every continent (bar Antarctica) is catered for, with over 46,000 positions in India analysed, along with 23,000 in Singapore and 7,000 in Brazil. It is an excellent tool to explore, but we took the time to look into the most important questions job seekers might have.
Which job is most in demand?
In the 2010s, data scientist was frequently listed not just as the most in-demand job in the data field, but as one of the most in-demand jobs, period. Yet Data Nerd's 2023 analysis throws up an interesting finding. Data Engineers are now more in-demand than Data Scientists. Here's the breakdown they found.

Clearly, while there are plenty of positions available in each of these disciplines, Data Engineers are now most in demand.
Just a word of warning on these numbers. For many companies, these roles are somewhat new. Plus, the landscape is changing quickly in terms of how different responsibilities overlap. As a result, companies are not always accurate in how they describe the type of professional they are looking for. You might be reading a job posting for a Data Analyst position and realise halfway through that the company is actually looking for a Data Engineer.
First of all, this means we should treat statistics on the number of positions available as general guidance rather than set-in-stone rules. Secondly, if you find a job description unclear, make sure during the hiring process that you are both on the same page to avoid disappointment later on.
Do I need a degree?
According to the job postings analysed by Data Nerd, roughly two thirds of the positions for Data Engineers and Data Analysts mention that a degree is required. But for Data Scientists, more than 9 in every 10 postings mentioned the need for a higher education degree.
Granted, only going on whether a degree is explicitly mentioned as a requirement in a job posting is not foolproof. It is entirely possible that, even if a degree is not listed as a requirement, recruiters will still favour candidates with a relevant higher education.
However, the fact that 33% of Data Engineer and 31% of Data Analyst job postings did not mention a degree is strong proof of a trend that industry experts have known for a while - degrees are no longer essential for landing a job. More hands-on forms of data science training, combined with a strong portfolio of projects, will often be looked on more favourably by employers than a degree.
What form of employment is most common?
Across Data Engineer, Data Scientist and Data Analyst positions, there was a clear preference for full-time positions over part-time or contract-based work. And less than 2% of the positions advertised were for internships.
While not entirely surprising, this finding does raise a significant question. If the overwhelming majority of job positions are full-time, how do students or rookies gain experience? Contractor work and internships are often the first step on the career ladder for new professionals, while part-time work can be a great way to study and gain real-work experience at the same time.
One solution to this challenge is to focus on training methods that replicate the work of real data science teams. By learning from industry experts, and building a portfolio based on actual work tasks, you can prove to employers you have the experience needed to take on your first full-time position.
Giedrius also recommends focusing on data analytics first if you do not yet have significant experience. "In many cases, if you want to get into data science but you do not have a relevant degree, it makes sense to go into data analytics," he explains. "You can then move into data science through self study and by looking for opportunities to do data science on top of your data analytics responsibilities."
What salary can I expect?
Overall, data science jobs are well paid, thanks for the strong demand for specialists in this area.
Beyond this general observation, however, it can be difficult to know what an appropriate salary is for the positions you are applying to. Given that you may well be asked what your expected salary is during the application process, this is an important factor to understand.
Data Nerd shares findings on average salary and median salary. While somewhat helpful, the average salary figures are distorted by the industry practice of putting very wide ranges for job postings. In extreme examples, they found job postings where the stated range started at $300,000 and went up to $750,000.
A more reliable data point is to consider median salary. Here are the median salaries per position according to Data Nerd:
$126,815 for Data Engineers,
$120,000 in Data Science,
and $85,000 in Data Analysis.
When researching for specific positions, use the country filter to get accurate figures for your region.
What skills are employers looking for?
This is the big one in terms of your long-term career development. You can dedicate months of your life to learning a new language or software stack, only to discover employers don't need these competences. So, understanding exactly which skills are in-demand is very valuable.
According to Data Nerd, skills like SQL and Python are more or less universally valued - both feature in the top 3 skills mentioned for Data Engineer, Data Scientist and Data Analyst positions.
For Data Analysts, knowledge of Excel, Tableau and PowerBI is sought after. For Data Scientists, Tableau is also important, along with R, AWS and Spark.
Here is a full breakdown of the top 10 skills in each role (correct as of June 2023 - Data Nerd's App updates all the time as new listings are posted).
SQL
Python
AWS
Spark
Azure
Java
Scala
Kafka
Hadoop
Snowflake
Python
SQL
R
Tableau
AWS
Spark
Azure
TensorFlow
Excel
Java
SQL
Excel
Python
Tableau
Power BI
R
SAS
PowerPoint
Word
Oracle
There is one more important point to note before you start learning some of the skills mentioned in this table. "Many of the skills shown in this table are alternatives to each other," Giedrius comments. "So a company will never expect a Data Scientist to know Python, R and Java - they will only be using one of these programming languages. So you definitely don't need to learn all of the skills listed here for a specific role - that would be very counterproductive."
Armed with this data, you now know which skills are required, what salaries you can expect and what kind of training you need.
So, the next thing you need to understand is how to find the perfect open positions to fit your personality and profile, and land an interview.
How to find open positions and land interviews
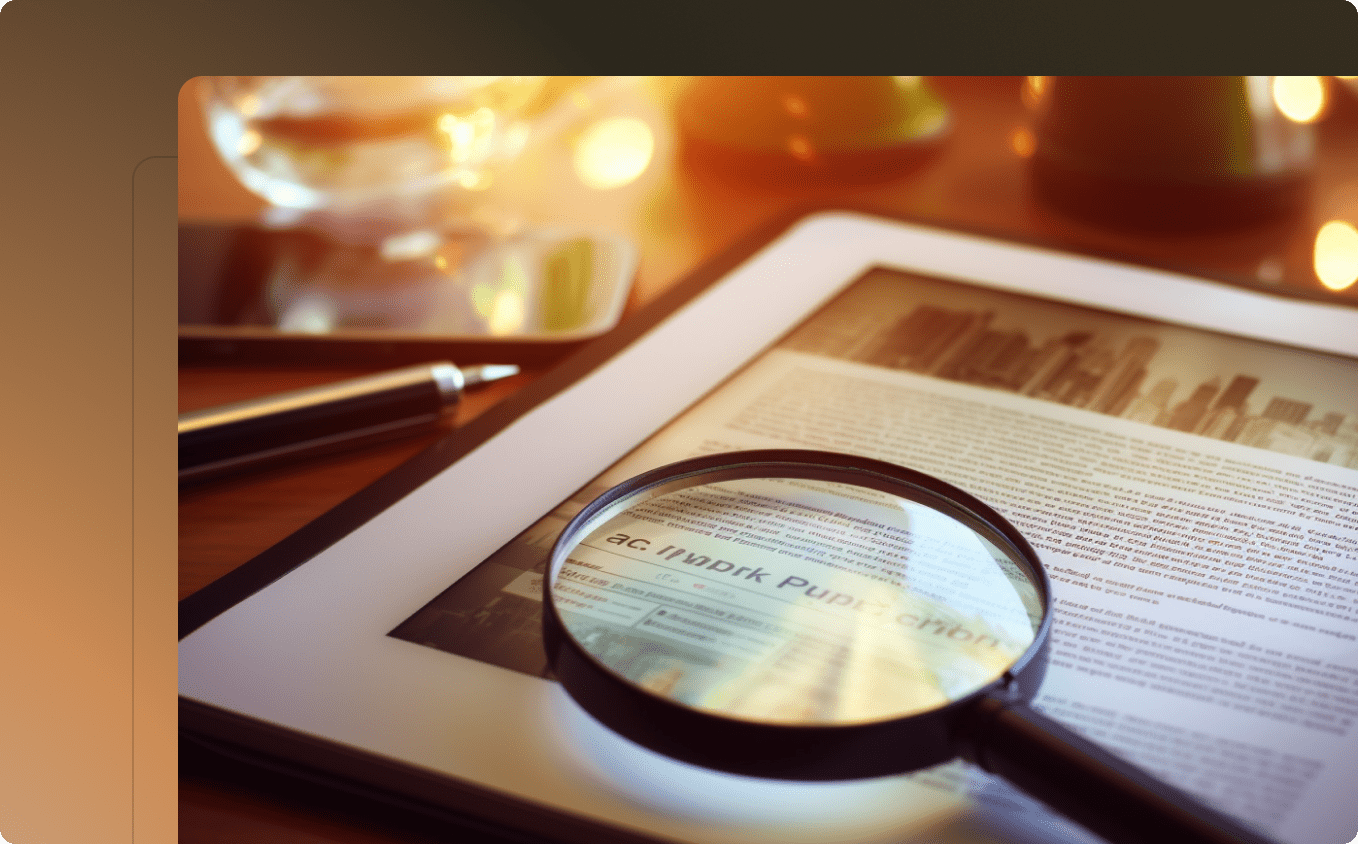
So you have a solid overview of the landscape, and understand what it is that employers are looking for.
Now it is time to start your job search. In this section, career coach and recruitment expert Simona Bareikė shares her tips on the best ways to find open positions. Plus, she has plenty of helpful advice on what to include in your application to help you get through to the interview stage.
The search: creating a job search system that works for you
When it comes to landing a data job, there really is no one-size-fits-all solution. There is massive variety when it comes to the industry, role, requirements and ways to connect with possible employers. "Along with the standard methods for conducting a job search, there are less conventional methods you can think about as well," says Simona.
The key principle is to evaluate what has worked for others, then use this knowledge to develop your own job-hunting formula. Naturally, a good deal of homework is in order. You will need to consider your current skill set, reasons for wanting a data job, desired impact and career path, the form of employment you prefer, and other factors relevant to you.
Speaking generally, you would do well to base your efforts on the following three principles:
Be consistent and seek to get multiple offers,
Conduct extensive research and curate a list of potential employers,
Go beyond conventional methods to connect with employers.
Consistency and seeking multiple offers
As a rule, those who follow a clearly-defined weekly job-hunting plan get hired up to 3X faster than candidates that don’t. Being proactive about submitting job applications to employers also greatly increases your chances of getting several offers simultaneously.
This, in turn, will result in a much stronger bargaining position. Keep in mind that companies often have a 15%-20% salary buffer when it comes to final-stage candidates. They can also usually sense whether you are desperate or not from the wording of your application message and your behaviour during the phone call or interview.
Having more than one offer will certainly help you, but there are still challenges when it comes to preparing for the dreaded negotiation over salary. In fact, this is something that around 55% of candidates don’t do. Here are some tips for approaching the topic of salaries:
Figure out the typical salary for your position and the company’s remuneration or career advancement system.
Also consider the benefits you get at your current workplace and compare them to those offered by the prospective employer – this should be a factor during the salary negotiation.
Finally, you don’t necessarily have to give a specific and fixed figure. For instance, you may be somewhat lacking in experience, so you might want to agree upon a lower amount, subject for review after a given period on the basis of the achievement of specific objectives.
In summary, submitting frequent, targeted applications will help you secure multiple options. And when you have these, you will be much less anxious to speak freely in interviews and much more capable of agreeing on a favourable salary. In fact, our experience with alumni shows that consistent job-seekers often end up with 2-3 times higher salaries than their counterparts.
Extensive research and curating an employers’ list
These days, “raw application” is no longer a very effective job-hunting strategy because there are often 100s of applicants for each position.
Instead, says Simona, "one of the key strategies in a job search is to compile a thoughtfully curated list of organisations that align with your interests and aspirations."
You can start this process by creating a "long-list" of companies that you have at least some interest in working for.
Next, “prune” your list based on extensive research into each one.
Don't forget, for positions as a data scientist or analyst, you don’t need to restrict yourself to the IT sector. From finance to agriculture to healthcare, there are a wide range of industries that need data scientists and analysts. If you have any prior experience in a given industry, this might be another promising avenue to explore.
"By carefully refining this list, it becomes possible to craft a compelling motivational message that highlights why you want to work for a particular organisation," explains Simona. "It also puts you in a strong position to show the value you can bring based on your prior experiences and qualifications."
With your list compiled and refined, you are ready to reach out to companies and organisations. Instead of mass emailing, Simona recommends a targeted approach. "Send personalised emails rather than relying on mass cold emailing," she says. "In my experience in HR, cold emails are usually treated as spam and can potentially do more harm than good."
"In addition to this, I would recommend exploring avenues to connect with your target organisations," Simona continues. "These could include meeting with potential managers or reaching out to HR personnel. This presents an opportunity to make a memorable first impression, as there might not be a second chance."
If a given company is fairly large (50+ employees), it is best to find the contact details of its hiring managers on LinkedIn and get in touch with them directly. On the other hand, if the company is relatively small, you may choose to connect with the data professionals on its team directly, as they will likely be managing themselves to a greater extent. And remember, when it comes to hiring managers, even if you ultimately get a negative response, they may still pass your resume on to their colleagues, potentially landing you an interview with a different company later on.
Go beyond conventional methods to connect with employers
Applying to open positions, sending out emails and connecting with managers and HR personnel are all important methods to use. But they are by no means the only ones.
"Along with these methods, I would recommend other, less conventional ways to search for a job," she comments.
"For example, I recommend participating in events like hackathons and other gatherings organised by data-related associations and communities. You can also attend conferences, take part in training programmes and explore tech fairs - these are all valuable avenues to pursue."
Although these methods are more long-term, they bring some specific benefits. "These activities are often supported by organisations who are looking to connect with the community and identify highly-motivated individuals," she explains. "So, while this approach may take longer, it can bring a wide range of unique opportunities that might otherwise not be available to you."
The prep: seeking clarification and understanding the position
When you find interesting open positions, taking care to understand exactly what is on offer is an important step.
Showcase you experience and qualifications when you connect with the company
As we have already shown, although a degree is not essential, data jobs typically require some qualifications, both academic and professional. And employers will also want an idea of your level of experience.
In other words, you will need to show: 1) what skills and qualifications you have gained, 2) real examples of the work you have done so far; and 3) what clients or employers have said about your work.
For starters, you should build a solid portfolio on GitHub and a strong LinkedIn account. Regarding your profile on LinkedIn, Simona has helpful tips to share:
"First, I would recommend analysing the advertisements for open job positions you're interested in. You can then start to systematically list the keywords found in these advertisements, and then search for these keywords. In this way, you will find more opportunities that are a good fit for you."
"You can also incorporate the same keywords into your LinkedIn account. This means LinkedIn's search algorithm will show your profile higher to people who are searching for those terms."
"Finally, I recommend focusing more on the "About" section of your LinkedIn profile - it usually doesn't get the attention it deserves. It is a very good place to reiterate the main keywords you want to be linked with, and to share your motivation for joining the data world. Naturally, your profile should also include details of your past experience, employment history, and your current skills."
Another tip is to use referrals to demonstrate that you are a valuable candidate and that your past clients and employers appreciate your work.
If you know anyone working for a company you are interested in, ask them for a short text about working with you. This will help your application, and has the additional benefit that the referee might inform you of opportunities that have not yet gone public. Developing an extensive industry network, while time-consuming, is necessary in today’s busy market.
Talk to recruiters (if they have the time)
Recruiters and HR heads are a very valuable resource when it comes to your application. They can clarify questions you might have about the role, and help you prepare effectively for interviews.
However, as Simona warns, many of these professionals are currently overworked, and reaching out to them prior to the interview probably will not yield a response. "It is important to recognize that recruiters and HR professionals, particularly in the aftermath of the COVID-19 pandemic, often face significant burnout," Simona explains. "Due to the high volume of candidates, they may not always have the bandwidth to provide feedback to every candidate after job interviews, so don't expect them to have time to educate candidates on interview preparation."
"Having said that, there are times in the process when reaching out to them might be worthwhile," Simona continues. "In my opinion, the optimal stage to seek clarification and pose specific questions is when recruiters or hiring managers reach out to you to invite you for a job interview. At this point, it would be appropriate to inquire about any additional preparation that could enhance the effectiveness of the interview process. Then during the interview itself, you can ask any remaining questions you have."
The pitch: applying for a job
In most cases, especially if you are competing against many other candidates, you will only have one shot at getting the hiring manager’s attention. This means that you have to make every word of your application count, says Simona.
"Each additional step in the recruitment process is an opportunity for you to distinguish yourself from the pool of competitors," she argues. "Despite the prevailing sentiment in the market that cover letters or similar messages are often overlooked, it is well worth seizing this opportunity to craft a compelling and impactful motivational message."
What should go into this message? According to Simona, your goal is to demonstrate that you are genuinely interested in working with the specific company you are applying to. "Your cover letter (and even your portfolio) should convey the idea that you are not simply applying to every position available. Instead, show that you are specifically targeting select opportunities with a tailored and distinct message."
So the overall goal is to write a cover letter that is laser-focused on the specific company and opportunity. But how exactly do you go about doing this? Here is Simona's step-by-step approach.
Research
"Before you embark on writing your cover letter or motivational message, it is essential to conduct thorough research. Familiarise yourself with the company and the specific job you are interested in. While carefully reading the job description is important, it is equally valuable to explore the company's website. You can also look through the company leadership's Twitter feeds, and review employee profiles on LinkedIn."
"This research will enable you to customise your message to reflect your genuine motivation. Perhaps you will discover a unique story or experience that resonates with the company. If not, consider how you can create a compelling narrative that is related to the interests and focus of the company or individuals."
Direct your letter to someone specific.
"Address the recipient directly and avoid generic salutations like "To whom it may concern" or "Dear HR." To do this, use LinkedIn or other channels to identify the title of the hiring manager and their name, the recruiter's name, or the specific team you are addressing in the company. Then begin your cover letter with a personalised greeting."
Include all of the relevant details needed
"While remaining concise, make sure you fit all of the relevant information about yourself in the message, as this may be forwarded to others. Give a brief rundown of your personal details: name, background, credentials, and profession (“I’m a data scientist/analyst”). Share a link to your final project, made available online. Assuming that you have about 20 seconds to make an impression, make the presentation easy to understand and, if possible – easy to share. Finally, give a quick but focused demonstration of your interest in, and knowledge of, the company you’re applying to and the industry they operate in."
Templates for demonstrating your interest in the company
The explanation as to why you are interested in a specific company will need to be written specifically for each new application. So, to make life easier and speed up your application process, here are 3 basic templates you can follow for this part of your application.
“I’m really interested in the problem of XYZ that [COMPANY NAME] has been working to address for the past X number of years.”
For example
"I have a deep interest in the challenge of digitising medical records in the developing world, which DigiMedRec has been working on since 2017."
👉 Elaborate on why these problems are important, what aspect(s) intrigues you the most, and how they relate to your skills and interests.
👉 Don't turn this into an essay. Your goal is to demonstrate that you have more than just a superficial knowledge of what the organisation does, and that you are interested in working on this or that problem. You can hint at your ideas for how to address these challenges, but there is no need to go into lots of detail
“[COMPANY NAME] has been on my radar for a while and the role currently on offer aligns with where I would like to take my career. I’m particularly impressed with [A RECENT DEVELOPMENT OR SOMETHING THE COMPANY EXCELS AT]”.
👉 Your goal is to be positive and show in-depth knowledge of what the company does. You don't need to go over the top with your praise - the most important point is to choose a concrete example of a recent development or company competence.
“I know that [COMPANY NAME] is recognised as one of the best places to work for Data Science / Data Analytics professionals. It has [MAIN POSITIVE CONTRIBUTION TO SECTOR] from the very beginning in [YEAR], and then grew by [PROCESSES THAT TOOK PLACE AFTERWARDS]. I am particularly excited about the way you have handled [SOME CHANGE OR PROBLEM] and am sure I could be of much help with XYZ."
“I know that DigiMedRec is recognised as one of the best places to work for Data Science professionals. It has been improving access to digital medical records from the very beginning in 2016, and then grew by offering micro-loans to help clinics cover their digitisation costs. I am particularly excited about the way you have handled complex and incomplete data sets, and am sure I could be of much help with improving the way insights are shared with medical practitioners."
Nailing interviews and tech challenges
.png)
So, your application stood out from the crowd, and you are through to the next round of the process. Congratulations! Now it is time to sharpen your interview skills and prepare for the tech challenge.
9 handy tips for interviews
Simona's years of experience in recruitment have taught her a lot about what it takes to have a successful interview. Here are her 4 top tips to help you succeed.
Know yourself
"Knowing yourself is very very important. You need to understand your strengths, weaknesses, motivations, and working style. You will also need to be able to recall real-life examples and successes from your previous work. To help with this process, I recommend gathering feedback from different people who know you well - friends, relatives, co-workers, and bosses. Seeking professional guidance from career coaches, counsellors, or mentors can also be beneficial."
Talk to industry insiders
"Before your interview, have conversations with professionals working in your desired field or positions. They can provide relevant insights on how to prepare for a job interview and what crucial information should be expressed during the interview process."
Recognize the value of transferable skills
"When changing careers, candidates often overlook their previous experience. This is a mistake, because this experience can provide valuable information to prospective employers. Therefore, highlighting transferable skills you have gained throughout your professional life is important."
Craft a tailored pitch
"This is a critical component of a job interview that sets the tone for the rest of the conversation and immediately demonstrates your understanding of the organisation and position you are applying for. Your pitch should convey all of the essential information about yourself within a concise and focused message. Similar to public speaking, it is crucial to "hook" the listener from the first few seconds in order to be heard effectively."
And here are some other general tips you might like to follow in preparation for your interview.
Review your resume
If you re-read your resume immediately before the interview then this information will be fresh in your mind and it will be easier to give specific examples. You will want to demonstrate your skills by bringing up real situations from the past to highlight your work style, motivation, strengths, and other characteristics relevant to the job.
Control your nerves
Interviews can be quite stressful, but you have to come in as relaxed as possible. This may sound banal, but one good way to calm your mind is by doing a bit of controlled breathing right before entering the door. Inhale for 5 seconds, hold your breath for 5, and then exhale for another 5. Do this several times and see if it helps. Many people swear by it!
Prepare your own questions
It is best to think of a job interview as an equal exchange, rather than a one-sided interrogation. So, when the time is right, ask your own questions of the employer. These could be related to the company's activities in general, or the specific role you are applying for.
Mention your interests and hobbies
This should, of course, be reserved for the later part of the interview. But it will help the recruiter connect with you as a real person, not just a resource for the company. In addition, having interests outside of work communicates that you are able to persevere and work towards self-assigned goals.
Get feedback
After the interview is finished, it is a good idea to ask for feedback, regardless of whether you end up getting the job or not. If the interview is successful and you ask for feedback, it shows that you have a growth mindset and are looking for ways to continually improve. And if you ask for feedback following an unsuccessful interview, you will have valuable information that can be a big help in your future career endeavours.
What skills should you mention during the interview?
Clearly, you will want the responses you give in your interview to directly answer the questions given. So, while it is always important to prepare, avoid scripting your answers beforehand. You want to be open, listening fully to the recruiter and specifically answering the question they give.
But instead of scripting, what you can do is targeted positioning. Here's Simona to explain more.
"When you are preparing for a job interview, you should analyse the job position. Do this in such a way that when you go into the interview you know which hard skills and soft skills they are looking for. In other words, you're saying 'OK, the emphasis in the job advertisement is on these X hard skills and these Y soft skills.' So this is what I'll focus on."
"Prepare yourself to present your answers in a way that covers these points. Of course, you should avoid lying or distorting reality, but I'm talking about targeted positioning which is very important. Your answers do not have to be scripted, but you must think through your overall strategy before the interview. This way, you can give a well-focused answer to a question like: 'What experience and personal qualities do you have that are necessary for this position?'"
This technique will work well for common open-ended questions used in interviews, for example:
Which professional skill do you most want to improve, and why?
Why are you interested in this position?
Give an example of a time you showed initiative.
Where do you see yourself in the future?
What are your biggest strengths?
Interviewers ask these questions because they want to see what your thought-process is like. When you have a wide range of possible points to discuss, seeing which ones you actually choose to focus on can tell them a lot. And if your answers match with the types of hard and soft skills they are looking for, you have a recipe for success.
Focus on soft skills
One important area to focus on when given an open-ended question is soft skills. In data science and data analytics, soft skills are just as important as technical skills. In fact, as much as 75% of long-term career success depends precisely on your soft skills. So, which soft skills should you emphasise? Here’s LinkedIn’s TOP10 in-demand soft skills in 2023:
Communication
Time and project management
Innovation
Intercultural sensitivity
Personal responsibility and initiative
Critical thinking, problem solving, and decision making
Thriving in a virtual environment
Resilience and integrity
Efficiency
Teamwork and relationship management
When it comes to data jobs, it is crucial to be able to present your findings in a way that is easy to understand for professionals and non-professionals alike. In other words, you must know how to explain data-based insights as a story or narrative.
So, when given open-ended questions during the interview, directing your answer to focus on communication, teamwork, critical thinking and personal initiative is a smart move.
One final point to emphasise is that most recruiters will use the interview to assess your soft skills and judge whether you will be a good fit for their team and culture. They will have a strong sense of your hard skills from your project examples, tech challenge and resume. So, be sure to dedicate time in the interview to focus on soft skills.
Preparing for the tech challenge
Information about the most common tech challenges is very easy to find on the internet. So to prepare, pick the position you are going for and identify the problems you may be asked to solve during the interview.
However, if you have experience of tech challenges in other fields, be prepared for a level up in difficulty, especially if you are applying for Data Scientist positions. The questions will be significantly harder than tech challenges or interview questions for Software Engineer jobs, for example. What the tech challenge covers will depend on the company's idea of the Data Scientist role. However, you can expect it to include:
statistics,
probability,
SQL,
software engineering,
and machine learning.
In terms of format, you should be aware that alongside standard tech challenges you may also be asked to complete a take-home technical assignment. This will then be used as the starting point in the tech interview itself.
Tips for success in your tech challenge
When tackling a challenge, here are some helpful tips that can improve your performance.
Ask questions after you receive the tech challenge
As Simona mentioned, once you have been invited for an interview or to take a tech challenge, this is now the perfect opportunity to ask for additional information. You may not always get a response, but it is certainly an acceptable thing to do. You could ask what the hiring manager's expectations are for this tasks, or if there is anything they would particularly like you to focus on. Asking will show you are taking the task seriously, and are motivated and interested.
A "README" file
Make sure you read the instructions carefully and then include a clearly written “README” file so that other developers could understand it without any need for additional explanation. It is also recommended to brush up on unit testing, as employers often look not so much to validate your code, but to ascertain your ability to write, test, learn, and iterate on it.
Be ready for feedback
After you are done, check for errors and then get prepared to receive feedback. This process is meant to test how you handle advice, criticism, and general input from other people. Do you steadfastly defend your every decision or are you able to react constructively and learn? The latter ability is obviously what employers prefer. So keep your cool, ask questions for clarification, and thank the recruiter for their input.
Think from the recruiter's perspective
The questions you will be asked during the interview and the tech challenge are often grouped under specific headings meant to assess your technical knowledge, practical experience, and communication skills. Keep this in mind and do your best to give answers appropriate to what the recruiter wants to find out.
Be honest
No one has all the answers to all questions, and employers don’t expect that from prospective hires. If you don’t know the answer to a given question, don’t try to fudge your way through it. Be honest, and show that you are willing to learn from your superiors and team mates, by making comments like: “That is an area that I haven’t had the chance to explore yet, but I would love to do so in the near future.”
The wrap up: getting hired in data science and analytics
From this handbook we hope you have gained a strong overall understanding of the types of opportunities available, what employers are looking for, and how to maximise your chances of getting hired.
Let's wrap things up with a couple of overall recommendations.
First of all, Simona stresses that when you are in the thick of a job search, remaining focused and organised is the key to success. "It is important to approach the job search process with a strategic mindset," she says. "Focus on quality over quantity when it comes to reaching out to organisations. By adopting a targeted approach and making a strong first impression, the chances of success in securing the opportunities you want are greatly enhanced."
Secondly, remember that personalising this process is very important. Hopefully, there are lots of useful tips and strategies you can adopt. Nevertheless, remember that each job search will be unique to the individual. Everyone has different priorities and needs, whether we are talking about employers or employees. So, be true to yourself in terms of which jobs you go for and how you present yourself. And remember to do everything you can to understand the other party's needs and tailor your applications and interview technique to suit them.
The opportunities in data science and analytics have never been greater, and there is plenty more growth to come. So focus on your goals, and you can be confident that you will land the right position for you.