How Data Analytics can benefit your business?
A guidebook
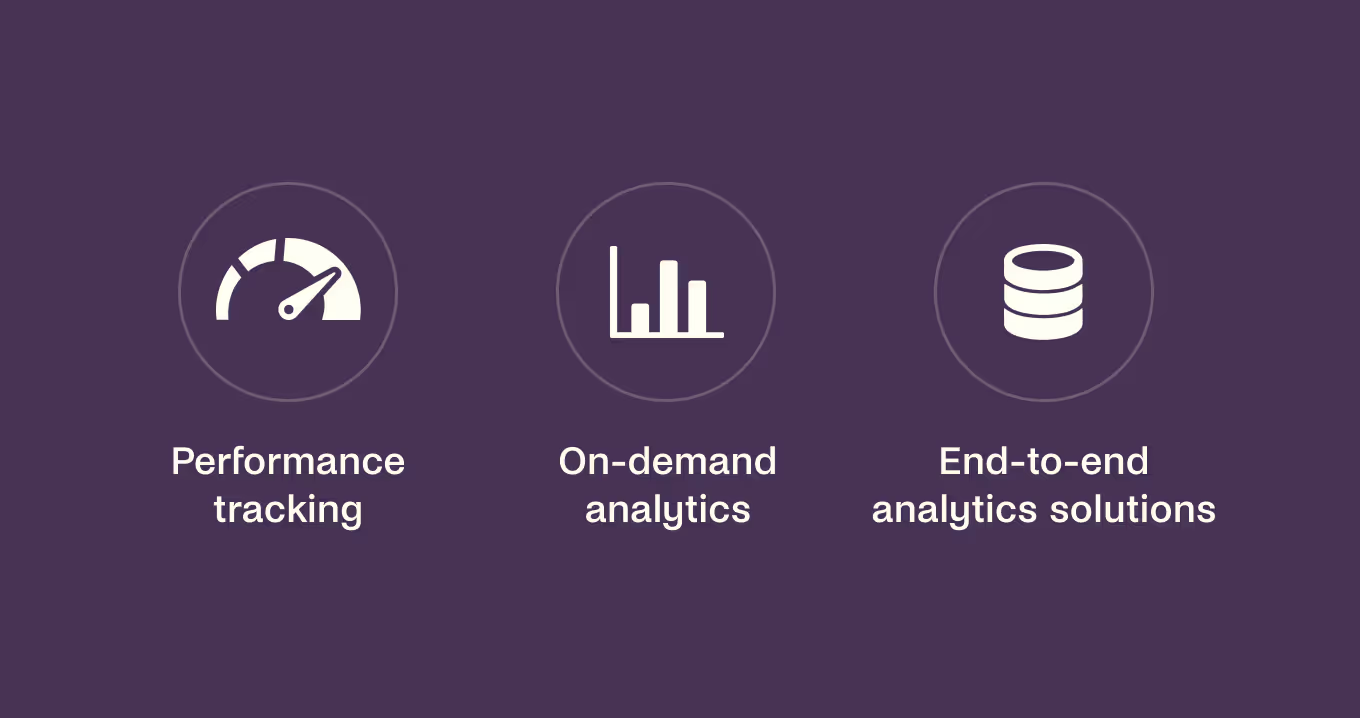
Data Analytics can transform your business in three fundamental ways. Firstly, by tracking performance you can get an accurate picture of your entire organization. Secondly, through on-demand analytics you can problem solve specific issues using data-based insights curated by your data analytics team. And thirdly, using end-to-end analytics you can proactively build data solutions that anticipate issues and provide not just a snapshot, but deep understanding. Creating and leveraging these advantages requires a Data Analytics team that is experienced, autonomous, and ready to work on building strong data foundations first.
This playbook provides an in-depth analysis of these advantages and sketches out how they could impact your business.
Introducing Data Analytics
In the 2023 business landscape, data is everything.
Digitization, accelerated by the COVID-19 pandemic, means companies are collecting data at a ferocious pace. While this data collection brings risks and challenges (cybersecurity, GDPR compliance, cloud and edge computing), these are far outweighed by the new opportunities created. To tap into these opportunities, you need Data Analytics.
What is Data Analytics?
In its raw form, data is a tangle of incomprehensible, meaningless numbers. The purpose of Data Analytics is to find patterns in this raw data and extract actionable insights. Put another way, Data Analytics is a type of business intelligence that uses the available data to gain a better understanding of a specific business area (e.g., customer behavior).
This process allows you to make sense of the past and make forecasts for the future across every part of your business: reach, revenue, operations, customer loyalty.
Why is now the time to embrace Data Analytics?
The days when business leaders could make decisions based on personal experience or gut instinct are long gone. Fact-based decision-making is the norm in today's leadership landscape. And it is evolving all the time.
Over the last decade, Business Intelligence (BI) has established itself as the cornerstone of this new type of decision-making. And Data Analytics tools are at the core of how BI works. Take Predictive Analytics, a form of Data Analytics where a company's existing data is mined in order to predict how decisions or trends might play out in the future. From chocolate bars to cars, Predictive Analytics has been fueling disruption and enabling outperformance for years.
But this is child's play compared to what is coming. AI and Machine Learning appear to be at the inflection point of their exponential growth. This means whole new ways of analysing data will emerge. It will also unlock access to previously impenetrable data sets.
About this playbook
We decided to put together this playbook to help business leaders and decision-makers understand, in concrete terms, how Data Analytics can benefit their business.
In light of the context we have just defined, it is our view that fully embracing Data Analytics is now imperative. But this can often lead business leaders to jump headfirst into practices and technologies they don't yet fully understand.
So, to begin at the beginning, we want to paint a picture of the different advantages Data Analytics can bring to your company. These are by no means exhaustive - that is not the point. Rather, they should help you quickly gain a clear perspective on the potential Data Analytics has.
The advantages
The playbook covers three fundamental advantages Data Analytics will bring:
Accurately tracking performance across your whole company,
Solve problems with agility using on-demand analytics,
Fully understand your customers and processes with end-to-end Data Analytics solutions.
These three advantages form a kind of pathway. For companies with little or no Data Analytics in place, advantage one (performance tracking) would come into play first, and form the foundation upon which other Data Analytics functions are then built.
Many of you will already have some forms of performance tracking in place. In this case advantages two and three (on-demand analytics and end-to-end solutions) should prove more valuable, providing you with an expansive view of what your Data Analytics team is capable of.
We chose this structure because it provides a logical (and somewhat chronological) way of understanding how Data Analytics can be baked into your everyday operations. But it is also important to stress that you don't need to take a linear, step-by-step approach to enhancing your Data Analytics. You can start incorporating elements of advantage two and three early on. Likewise, you will want to keep revisiting and refining what is happening with advantage 1.
Finally, after exploring these advantages in depth, we share some tips on how to build and grow your Data Analytics team so it is hardwired for success.
Expert insights
This playbook includes extensive insights from two experienced data specialists. Dominykas Balčiūnas is a Data Project Manager at Kilo Health, one of Europe's fastest growing tech companies with over 4 million active users. His main focus area is data projects that span the whole organization - data warehouse development, key reporting revamp, as well as data observability implementation. Dominykas is experienced in working with big data analytics and analytics engineering.
Andrius Didžiulis is Head of Data at BARBORA, one of the largest grocery delivery services in the Baltic States. His expertise is in planning data projects, building analytics systems and predictive models, and managing Data Science teams.
Advantage 1: Accurately track performance across your whole company

Data Analytics can facilitate a substantial improvement in a fundamental area of your operations - tracking performance. This improvement enables better decision-making and greater business agility.
What is the situation before you incorporate Data Analytics?
With little or no Data Analytics activity in place, a typical company will suffer from 3 interconnected problems.
Skilled data and inconsistency across teams and departments. Data Analysts are the people in your organization who think deeply about who has what data. Are different departments collecting different sets of data? When and how is this data being shared? These questions are fundamental to understanding what is going on within your organization. For example, you want to understand your company's impact on the environment, and then track it over time. First, you're going to need each of your teams to gather data in a consistent manner so it can be pooled. Then, you're going to need them to share with one another so teams have a yardstick by which to evaluate their own performance.
Unaligned stakeholders with different understandings of what is happening. When your company's data is uncollated and unstructured, it is easy for misalignment to spread. People and teams within your organization will reach their own interpretations on what your data means. Or even worse, they will ignore it completely and rationalize decisions based on gut instinct or because it's "the way we've always done it." This is bad enough when one person is doing it. When you have multiple stakeholders reaching decisions in this way, it is a recipe for chaos, errors and exclusion, with those who shout the loudest normally getting their way.
Inaccurate or missing data for specific KPIs. If you're like 99.9% of businesses out there, you place huge stock in your performance metrics and KPIs. But how accurate are they? Is the data you're working with reliable? Are there gaps in your dataset that give a false impression? This goes to the heart of your whole decision-making process. And without effective Data Analytics in place, these question marks will always be there.
These problems all add up to one result - a lack of understanding and poor decision-making. Dominykas puts it simply: "You will struggle to know what the macro trends of your business are, or understand why they are present."
What is the situation after incorporating Data Analytics?
Hiring Data Analysts and setting them to work on your performance tracking will lead to a more integrated and accurate set up. Better still, every stakeholder will be able to better understand their situation and make appropriate decisions.
Integrated data that facilitates a macro perspective. "With Data Analytics in place, you will be able to report unified metrics across the company," explains Dominykas. This means all of your data is in one place, so you can build reports and track performance. "Once you have your data unified, you can automate reporting - we have automated Tableau reports that track our main KPIs," explains Andrius.
Unambiguous data that creates company-wide alignment. Embracing Data Analytics also means reducing ambiguity. Not only will your reporting now be built on a unified and coherent data set, it will also be easier to make sense of. "Data Analytics helps you align your stakeholders," Dominykas points out. "You get clear KPIs connected to a single goal, and the data presented in a format that is easy to understand for seniors and juniors alike."
Reliable data you can base decisions on. Data Analysts also inject added confidence into the tracking and reporting process. Knowing that the data is reliable and accurate will help engender decisiveness within your organization.
Why does this improvement matter for your business?
Put simply, using Data Analytics to enhance your performance tracking facilitates understanding, as Andrius explains: "It enables you to understand your processes and your clients better, and to get more value from what you do."
"It also helps you to make more confident decisions," Andrius continues.
"This improved decision-making brings (at least) 3 significant advantages, says Dominykas." You get:
better planning and resource allocation,
more predictable growth and development,
and improved strategizing.
How do you use Data Analytics for performance tracking?
3 practical tips
The advantages are clear. But once you have resolved to use Data Analytics to help with performance tracking, what can you do to ensure it goes as smoothly as possible? Dominykas has 3 tips.
👉 Tip 1: Get clarity from the main stakeholders on what to track before you begin
"Data Analysts should mirror the needs of the company. It should not be a case of 'I think it would be useful to track this.' Instead, it should be 'What do key stakeholders in the company actually see as being useful to track?'"
"So, before you start with any Data Analytics activity on your performance tracking, you need agreement from your stakeholders as to what needs tracking. Data Analysts should gather information from everyone who has a decision-making role, and ask them: 'What do you need to track, and how can this data be used to benefit you?' Everything that is developed should have a clear answer to these three questions - “Is it relevant?”, “What problem does it solve?”, and “Is there anything more useful I could do?.”
👉Tip 2: Be rigorous on data quality and modeling
"Performance tracking will usually be the first step in your company's Data Analytics journey. So, this is the moment to really focus on data quality and accuracy. Mistakes made at this point might affect all of your other Data Analytics activities in the future."
"Data Analysts should set up a monitoring process for checking data quality, and take time to model the data correctly."
👉 Tip 3: Think LEAN and prioritize clarity
"I've always liked the principles of LEAN, and I think they apply well to Data Analytics. It's like the Pareto principle - you can achieve 80% of your goals with 20% of the effort. But for this to happen, you need clarity from your main stakeholders. In the case of Kilo Health, our mission is clear and everyone is onboard. In these conditions, it's easy to know where we are going and what kind of tracking we need to develop. Basically, you know what you're doing."
Advantage 2: Problem solve with agility using on-demand analytics
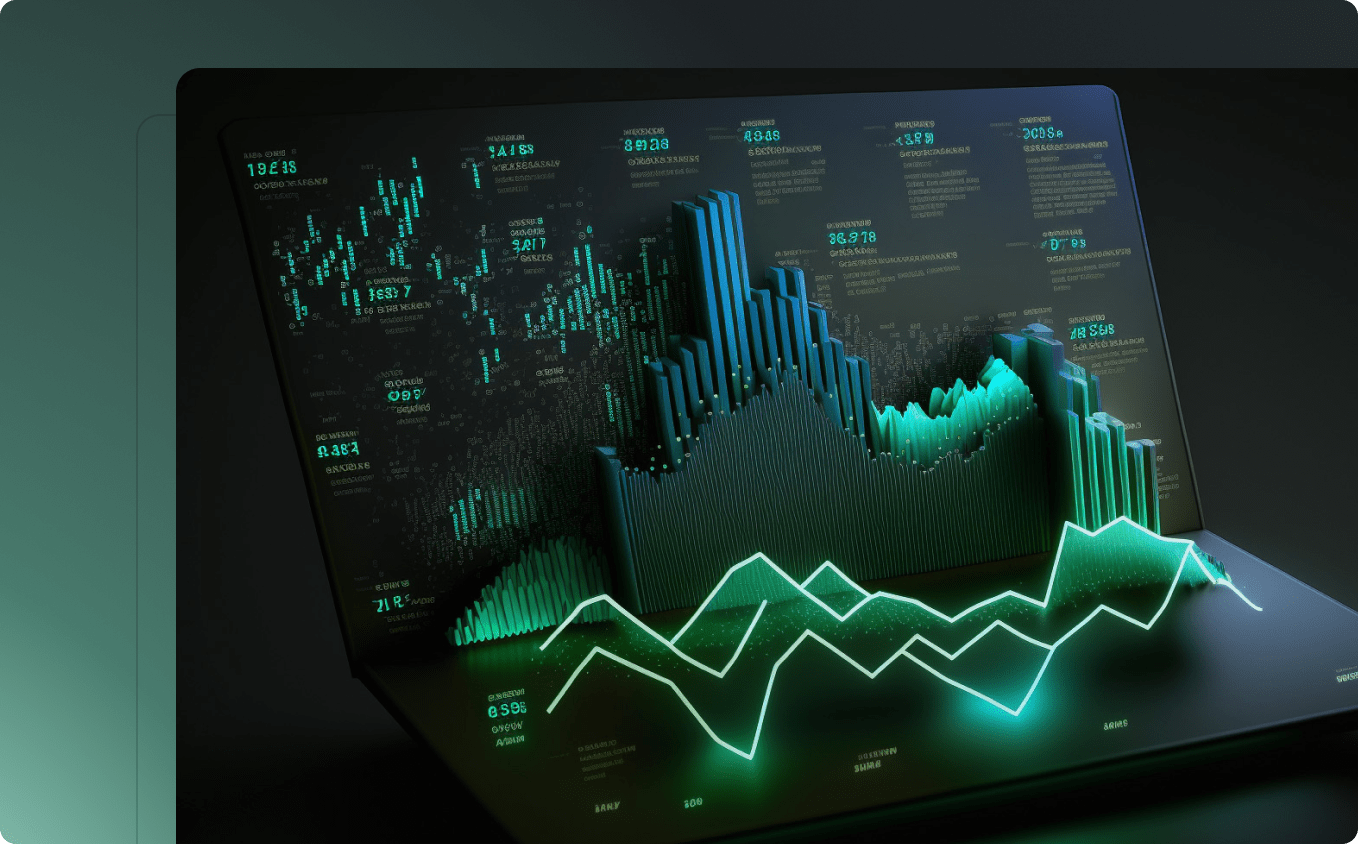
Fast, agile decision-making on complex business-critical questions. On-demand Data Analytics offers just this, with stakeholders coming to the data team with specific requests and receiving bespoke analytics that help them quickly arrive at the right conclusion.
What is the situation before moving to this stage?
On-demand analytics is the next step up in terms of Data Analytics value for your company. You may already have scheduled performance tracking in place - in other words, a reactive setup where you have plugged in specific KPIs and you generate reports on them at specific periods (weekly, monthly, quarterly).
But this leaves plenty of gaps that still need filling. What about questions that occur in the periods in between your scheduled performance tracking? What about issues that aren't covered by your KPIs?
For these, it is usually up to product managers, sales leads, CMOs and whoever else to make decisions. And the degree to which these decisions are data-based is likely to vary substantially. The result? Inconsistency, confused processes and a lack of direction.
What is the situation after incorporating on-demand analytics?
The most obvious - and perhaps most significant - change is that decision-making will become more data based. This is especially important in environments where the decisions needed are frequent, high-speed, and complex.
"On-demand analytics is hugely valuable to companies that are already well-established," says Dominykas. "These companies typically need quick decisions, and they often face situations where there is no 'right answer.' So the ability to go to a Data Analyst and say, 'Here's my problem. Can you look into the data to help me make a quick decision?' is very valuable."
Furthermore, this type of Data Analytics can be set up ahead of time as well. "A stakeholder can come with a general question, and the data team can set up monitoring. Then they can alert the relevant stakeholder when there is an important development, change or anomaly that might need to be addressed."
And this hints at a second important benefit - confidence and peace of mind. "On-demand analytics makes life easier for people in your company who are responsible for a lot of different issues and priorities. Now they have peace of mind that they can get valuable insights quickly, or have their most critical areas monitored," explains Dominykas.
Finally, with this approach, the use of data within your company becomes more granular. Rather than just tracking the biggest KPIs, you can zoom in on micro topics. "It allows you to investigate a specific question. For example, 'Why did the refund rate drop?'"
Why does this improvement matter for your business?
Andrius has a case study that neatly illustrates how valuable on-demand analytics can be.
"We were thinking about the pricing of delivery for our products," he explains. "The goal was to gain additional income through added sales without losing too much in delivery costs. So our Data Analytics team ran an analysis. They looked at how many purchases and how many customers would be affected by the changes we were considering. And they came up with a range of scenarios, from best case to worst case."
"This enabled the stakeholders to make a well-considered choice on delivery pricing. Later on, we checked how the number of purchases and customer behavior had changed, and it matched with our forecasts."
Here's a summary of how that process worked:
the decision-makers came to the Data Analytics team with a highly specific question on a business-critical issue.
The Data Analysts dug into the data to provide insights on what could happen if different options were chosen.
This empowered the decision-makers to make a fast choice on a highly complex topic.
The Data Analysts were proven right, and this confirmation helped them to further refine their analytics.
Here we see Data Analytics moving beyond mere tracking into providing bespoke advice. "If the data team receives a question, they can prescribe a specific suggestion," explains Dominykas. "For example, let's say the refund rate grew by 5%. We can use the data to suggest a course of action, like targeting a different demographic or running ads on alternative channels."
This means that, along with the ability to make better-informed decisions, stakeholders also have greater confidence in their overall processes. They know that certain metrics are being tracked and they can quickly get detailed insights on bespoke questions, as and when they arise.
How do you set up on-demand analytics?
2 practical tips
On-demand analytics is a more complex service than performance tracking, and its success hinges on your team's understanding of how data works. Here are Dominykas' tips on how to do it right.
👉 Tip 1: Get the whole company onboarded
The on-demand model places a lot of onus on various stakeholders within the company to proactively approach the Data Analytics team with questions and topics. So, perhaps the most obvious pitfall is that team members simply don't make use of this option. Perhaps they think the data won’t cover it, or that going into the data will take too long.
To avoid this, a critical first step is to make sure that everyone who will be making decisions is familiar with what your Data Analytics team is doing, and can do. "It's very important to have the whole company onboarded and aligned," Dominykas points out. "They need to understand how performance tracking and other data services are being developed. And they need at least a sense of who will be involved (in terms of departments and data sources) in order to answer a particular question. This means they will already come with clear expectations and a good understanding of how their question will proceed."
👉 Tip 2: Get your data team to educate the rest
Dominykas' second tip aligns closely with the first. If more of your team are spending more of their time making sense of data, it makes sense to equip them with the tools they need. And your data team are the best people to handle this.
"The data team should educate stakeholders so they understand what value the data can bring," he explains. "They need to know how to ask the right questions and how to read the data that is generated. If you haven't been educated as to what the data can do, you probably aren't going to come up with a bunch of questions that are very good in terms of the company moving forward."
By contrast, educated decision-makers combined with on-demand analytics and monitoring can move things forward very quickly indeed. "When you have the combination of an educated workforce, monitoring and on-demand requests, what you get is very dynamic and fast-paced Data Analytics that’s no longer a bottleneck."
Advantage 3: Move from seeing to understanding with end-to-end analytics solutions

Empowering your Data Analytics team to proactively build solutions and solve problems will enable your organization to gain a deep understanding of your processes and customers. As your Data Analytics moves from describing situations to explaining them, you will level up your decision-making and strategic planning.
What is the situation before moving to this stage?
When tracking and on-demand analytics are set up, you are ready for the holy grail of Data Analytics - end-to-end solutions.
Prior to this stage, the onus has been on the stakeholders and decision-makers within your company to define how Data Analytics is used. They choose what is going to be tracked. They select the questions that data will find answers to.
"The Data Analytics team takes a reactive role," Dominykas points out. "They're either tracking metrics periodically, or acting in response to questions and requests. They might set up alerts, but even then they are still essentially being told what to track and monitor."
While this setup is adequate in most instances, it results in missed opportunities. What other topics are there that would benefit from Data Analytics? Are requests being initiated too late? Is there a different way to look at certain decisions or strategies?
These questions, and many others, can be answered by a Data Analytics team with thorough coverage of the relevant topics and the license to propose solutions and initiate activities.
What is the situation after incorporating end-to-end?
"When you move to an end-to-end setup, your Data Analytics team becomes a proactive solution producer," says Dominykas. "They no longer wait for direction, but come up with suggestions and ideas themselves."
This could mean proposing new KPIs to track, or suggesting tweaks to existing practices to optimize performance. The team could even come up with more radical suggestions that impact the company's overall strategy and direction.
This type of proactive input becomes possible when the Data Analytics team has sufficient coverage of a topic or area. "When you can monitor the refund rate, for example, and have analyzed certain issues about it, you're then in a strong position to make suggestions. You can explain why it drops or increases, and this explanation will imply certain changes or actions."
And the potential output of this proactive form of Data Analytics is only set to grow, says Andrius. "The number of applications for Data Analytics has grown exponentially in the last couple of years," he explains.
Why does this improvement matter for your business?
For Dominykas, the critical change that occurs when you achieve end-to-end solutions is that you move from describing to explaining.
"When someone comes with a question, you can consult the data and model the outcomes, then pick the right one," says Dominykas. "But, have you truly understood why?"
"With tracking and on-demand analytics, you can very accurately describe what has happened already, and what might happen if you make certain decisions. But end-to-end gives you the possibility of explaining it because now you actually understand what is happening."
With this understanding, it is possible to create totally new solutions for your processes and systems. "Your data team is now in a position to create a whole product that runs by itself."
How do you set up end-to-end analytics?
2 practical tips
Unsurprisingly, achieving end-to-end solutions with your Data Analytics requires time, resources and focus. Dominykas has two tips to help you along the way.
👉 Tip 1: Employ Data Engineering practices
"When you're building solutions, it's important to start employing Data Engineering practices. It's common in Software Development to use Software Engineering, and Data Engineering is becoming increasingly common in Data Analytics. This engineering includes scheduling models that run correctly, and considering how the data is used and who the end user is."
👉 Tip 2: Nurture your team to keep it intact
"The success of end-to-end solutions is fundamentally connected to the people developing them. This means you need to form a very strong team, because they will be developing these solutions for months or years."
Assembling a data team and setting it up for success
To tap into the transformative advantages of Data Analytics, companies will need to build Data teams that have autonomy.
Dominykas has some valuable tips on how to put together your Data Analytics team, and what steps you should take to ensure it is able to operate effectively.
👉 Tip 1: Look for people who are happy to be out of the limelight
"If you're working on end-to-end solutions in particular, you have to be prepared to be in the shadows. There might be 5 hours of your working week where you are visible - the rest will be spent doing research, writing code and developing solutions."
"So when you're recruiting Data Analysts, look for people who will thrive in this kind of set up. If they need constant recognition and a high profile all the time, it probably won't work."
👉 Tip 2: Look for people with proven track records, them give them freedom
"It's important to recruit Data Analysts who have proven they can build the solutions you need, and that they are motivated to just code."
"But once you have established this, give them freedom to explore. They will need time and resources to achieve a good outcome - it won't work if you just say: 'here are your tasks - you have 10 hours to complete them.'"
👉 Tip 3: Let the Data team take on its own character
"I would recommend treating your Data Analytics team differently to a standard operational team. Let it develop its own values and core, and its own approach to decision-making."
👉 Tip 4: Empower decision-makers from your Data team
"This decision-making should extend beyond the Data Analytics team. If you're serious about integrating Data Analytics into your company's operations, Data Scientists and Decision Scientists should be empowered to make decisions at a company level. And for initiatives directly related to your data activities, ensure they are led by Senior Data Scientists who have buy-in from management."
👉 Tip 5: Establish strong foundations first
"There is a lot happening in the Data Analytics field. For example, the data mesh concept is very popular now. Google, with GCP developments, and AWS are bringing out new innovations all the time."
"In this environment, it is tempting to always think about the future, or get excited by the latest breakthrough. But I would stress the importance of focusing on the foundation of your data - the quality of the data, the data architecture - first. Make sure the most important steps are done correctly, and then you can start to explore the latest solutions.
Wrap up
The "before and after" for data analytics is dramatic.
Before, businesses struggle to track and share unified metrics across their organization. Decisions are slow and often based on sentiment or previous practices, rather than concrete data. They also tend to be reactive, putting companies on the back foot.
After, organizations benefit from a clear set of unified data that they can track and measure to gain an accurate understanding of macro performance. Stakeholders can now gather comprehensive datasets tailored to their needs or issues on which to base their decisions. And the data team offers preemptive insights, and even takes decisions, using systems they are constantly building and refining. And this means companies are out in front of any problems, responding early when fixes are cheaper and less-time consuming.
The volume of data is only going to increase. The same goes for AI and Machine Learning systems that order and analyze this data. Which means we have only seen the tip of the ice-berg in terms of Data Analytics' potential.
Make Data Analytics your new superpower
It is easier than you would think to add the Data Analytics skills and competences needed to realize the benefits mentioned in this playbook. Turing College's self-paced, learn-by-doing platform features a curriculum developed in partnership with tech companies. This means it offers a flexible and effective way to acquire real-world Data Analytics skills, whether it is for yourself or your team.